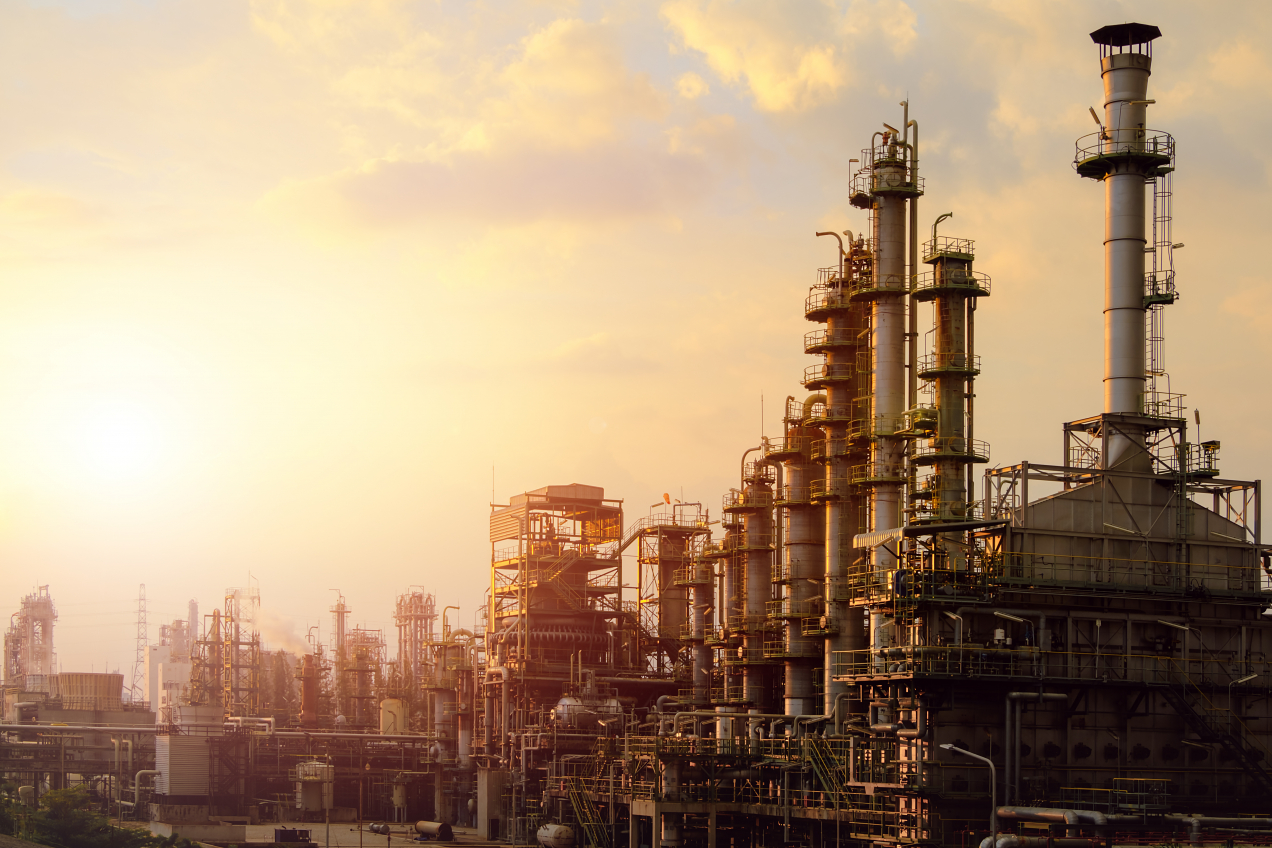
The Problems of Aging Plants
In North America, many oil refineries and chemical plants are close to 100 years old. In the EU, many plants were re-constructed in the 1940s and 1950s, making them 50 to 70 years old. These plants were built with little or no automatic control, and up to the 1970s, were run manually or with rudimentary control loops. In the 1970s, process plants began to install computerized control systems on some processes. Also in the 1970s, many manufacturing plants began to install programmable controllers to replace hardwired relays on assembly lines. These tools were employed to improve throughput performance in the plants and improve repeatability in quality.
Beginning in the 1980s and 1990s, the people who originally designed, built, and operated those plants were beginning to retire. These people were experts on their plants and their products and processes, and in the words of a plant manager in the USA, “My expertise leaves the plant every day at 4 PM and some of them don’t come back. They don’t leave their expertise and institutional knowledge—they take that with them into retirement.” The good news for plant management is that because of a number of recessions and stock market upsets, and because of Covid-19, the retirements of these aging experts have been somewhat delayed. But the Baby Boomer generation is now aged and retiring faster than they can be replaced. In addition, over 1 million people have died of Covid-19 so far, including many people whose expertise has suddenly been lost.
As the experience and expertise drain continues to occur, the plants continue to get older, and the infrastructure continues to be, in a word, rickety. Originally, maintenance was done when something broke. This often meant that the process or production line would stop until the problem was fixed. By the 1990s, it was no longer possible to do that. Maintenance went to scheduled downtime, when the plant could be shut down so preventive maintenance could be done. This didn’t always work because equipment did not always last until the orderly shutdown could happen. Worse, equipment doesn’t always break down completely—just degrades in performance until it no longer works. In addition, doing preventive maintenance on high value assets may be increasingly costly, especially if it isn’t necessary. While you are repairing the main inlet pump of your refinery, you aren’t putting crude into the plant, and you aren’t making product. If it isn’t necessary to repair or replace it, you are losing money.
“Because of the loss of expertise in operations, you need new, data-driven maintenance strategies, in order to continue operation. Especially if your management wants you to run the plant at higher than design capacity, and far longer than its expected life.”
In fact, you need process data beyond process control. You need to be able to access the meta data about your physical plant, rotating machinery, vessels, and process equipment. This is the basis of Industry 4.0 maintenance strategies. Once you get access to this data, you can also use it for process optimization, work process optimization, and even energy management. The data coming from machinery and even your vehicles is critical to be able to maximize your return on investment by timely maintenance.
Companies are turning to digital technologies such as IoT (Internet of Things) sensors in addition to process sensors and using predictive analytics to turn this data into real value for the enterprise.
This enables you to be more in control of your aging assets and reduce your unexpected outages. And it enables you to move beyond the old economy into the new digitized economy where one of the major products of the plant is data. The idea is that you can process this data with predictive algorithms which can determine when assets might fail.
What to Do With Multiple Generations of Systems and Assets
The older systems in your plant are usually reliable because they have been performing for many years. But they can also fail, and they often fail unexpectedly and catastrophically. And they are usually built up of layers of new construction. For example, when a new control system is installed, the wiring to the sensors and control valves is left in sit and married to the new control system’s marshalling cabinets, or from the existing marshalling cabinets to the controllers themselves. Sometimes there can be three or four generations of wiring and data highways.
“Sometimes there can be three or four generations of wiring and data highways all wired together.”
Stand-alone CMMS and EAM systems also collect vast amounts of data which is often siloed and not easy to use for process optimization or to optimize maintenance operations. More modern process instruments and programmable controllers have digital outputs. HART, DE, Profibus, Profinet, Fieldbus, and Modbus all are legacy outputs from instruments, control valves, and controllers. Often the data that could be accessed from these instruments and controllers is ignored or forgotten. But not only can you get the measured Process Variable (PV) from these devices, but calculated variables, instrument health reports, and other data that will improve the operational understanding of the plant and its installed base.
But if you can gain access to this data, and be able to use it, you will have a far better understanding of the health of the installed base in your plant. This understanding can lead to improving maintenance costs, scheduling, downtime, and repair/replace business decisions.
“Working with the additional data you can get from your existing plant can allow you to optimize the maintenance processes and reduce downtime.”
Working with the additional data you can get from your existing plant can allow you to optimize the maintenance processes and reduce downtime. By improving the equipment health of the plant you can also boost utilization and productivity, improving quality of production and the entire customer experience.
How to Get To Industry 4.0
Industry 4.0 is also called “smart manufacturing.” It is smart because it is made up of smart devices, smart instruments, smart machinery, and smart control systems all working together with a smart supply chain.
In order to make and keep your plant smart, you need intelligent Asset Performance Management systems. Companies have been trying to put together workable asset performance management systems for decades, starting with paper-based systems. These systems generated reams of paper but the paper was hard to store, and hard to search and was often used as doorstops in control rooms and engineering offices.
Next, computerized asset management systems for the enterprise (EAM) and Computerized Maintenance Management Systems (CMMS) were used to displace paper maintenance systems. These systems were generally stand-alone, and often hard to use and keep updated.
Next came condition-based maintenance tools and the concept of reliability-centered maintenance. Along with defining the asset strategy, this is classic specialized APM.
Fourth generation Asset Performance Management includes functionalities from the plant floor and the process controls to the business systems, including CMMS and EAM systems. But this is all built on a framework of big data analytics that includes descriptive, diagnostic, predictive and prescriptive analysis. That means the analytics can tell you what happened, why it happened, predict what will happen, and prescribe the correct actions to take to solve the problem. It isn’t limited to describing or understanding past behavior of the systems in your plant. It can actually be used to see what is happening and predict what to do to solve the problem.
“Predictive analytics is considered state of the art, but fourth generation APM will actually take you to a higher state: prescriptive analytics.”
Predictive analytics is considered state of the art, but fourth generation APM will actually take you to a higher state: prescriptive analytics. If you can predict what will happen in your plant, and you can do it accurately, repeatably, and with enough lead-time to give yourself options to deal with the problem, you can maximize uptime, maximize throughput, and even become more agile.
To make this work, you need to develop a “digital twin” of your plant and its processes. This digital twin is a high-performance software model of your plant that operates in parallel with the real assets of your plant in real time. The digital twin gathers data and updates itself continuously with new information about the actual assets, the system, and external data sources. The digital twin analyzes data using machine learning and other AI techniques. The twin looks at historical data, current data, and multiple simulations to determine what might happen, and the relative risk and confidence factors associated with options going forward. The twin creates predictive models designed to predict asset failure and breakdown.
APM can be deployed on a chip, for installation into a smart device.
It can be deployed on edge computers or to cloud infrastructure connected to enterprise sites or to plants. Because it is based on Industry 4.0 technologies, APM has easy connectivity to existing CMMS/EAM systems like SAP, Ultimo, Maximo, and others.
“We believe that the best way to provide Industry 4.0 APM is as an agnostic system, capable of connecting to all data historians, such as PI, AspenTech IP21, Yokogawa ExaQuantum, Honeywell PHD, and many more,” says Oudmans. “We can use our extensive knowledge of critical parts in industry assets to turn them into smart objects.”
UReason’s approach to APM is designed to be operated by end users with no great data science capabilities.
“We think that our no-coding approach gives the domain engineers the ability to reason using the streaming asset data,” Oudmans says. “So we have created an intuitive interface that you can use to set up your application or solution. UReason’s APM software is designed to integrate with existing CMMS and work-order systems.”
According to UReason, they’ve worked with hundreds of clients, successfully setting them on the road to Industry 4.0.
Download E-book
Users should be able to use next generation Artificial Intelligence (AI) technology for Asset Performance Management by themselves
“There are only three operations drivers really,” says UReason co-founder Jules Oudmans. “First, generate more revenue through agility and increased throughput; second, lower the costs of energy, regulatory compliance, unplanned outages and asset failure; and third, reduce risk.”
UReason is a company based in the Netherlands. “Our company was started in 2001 by some industry pioneers,” Oudmans says, “with the idea that asset management did not have to be difficult. Our belief was that users should be able to use next generation Artificial Intelligence (AI) technology for Asset Performance Management by themselves without having a complex and cumbersome process or methodology. And we were right.”