Here are some steps for you to take to begin the journey to prescriptive maintenance. First, it is a really good idea to make sure we know what prescriptive maintenance actually is.
Preventive maintenance has been developed over the past several decades to attempt to catch failure in the act, and fix problems, hopefully, before they occur. Predictive maintenance can help to bypass impromptu corrective maintenance action by trying to predict when a failure will occur, and what kind of failure mode it will be. This way you can schedule maintenance before the failure event and keep the process working with minimal downtime.
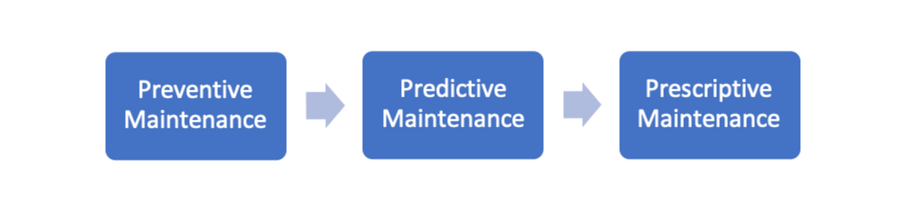
Predictive maintenance technologies and models have advanced significantly through the use of IIoT sensor technology and AI decision making software. Once you are able to predict with very high accuracy the mode and occurrence of failures, you can shift to prescriptive maintenance. Prescriptive maintenance technologies go further by looking for the signatures of failure and providing information on how to minimize or avoid downtime caused by unplanned failures using algorithms and Artificial Intelligence to extrapolate from a large amount of historical data and allow simulation and risk assessment to be done in the model, not in the physical asset.
Starting with what you have, make an inventory of the assets you want to subject to prescriptive maintenance. If the asset is complex, break it down into the smallest components that make sense. Using UReason’s APM Studio makes it easy to list inventoried assets and components on Asset Cards, like digital Pokémon Cards.
Let’s say you are going to initiate prescriptive maintenance on a pump. First you need to gather together all the information you can get about the pump, the application, and the baseline performance information, and input all that information into the model. You need things like flow, total dynamic head, head, efficiency, energy consumption, and more. This allows you to look at the baseline performance of the pump as an asset.
Then, you construct cause/consequence models, like bow-tie models, so you can start with an unwanted event like cavitation. That goes in the center of your model like the knot in a bow-tie. To the left, you enter the causes of that event, and you can concatenate those causes. For example, “static head too low” leads to “low suction pressure.” On the right side of the event, you start entering the consequences of the event. So, “duration of cavitation” leads to “lower operating regime” leads to “pump failure.” You can concatenate consequences, too: “pump failure” could lead to unit shutdown or other additional consequences.
UReason’s implementation of cause/consequence models is low-code and simple enough for engineers and operators without significant experience in data science to build and operate.
UReason also provides for dashboard visualization. These dashboards can be configured by role, so that engineers and operators and maintenance technicians see the dashboard that is best optimized for the information they need.
Once you have gotten all this information into the model, APM Studio helps you develop a digital twin which can be trained to accurately predict failure modes and events, allowing you to reduce unplanned outages to near zero. Being able to predict failures allows you to prescribe better operating modes, earlier maintenance cycles, and so you have implemented prescriptive maintenance on this pump asset.
This “pump digital twin” can be merged with other digital twins in the plant to do the same exercise: define the asset, design the cause/consequence model, and train the model to accurately predict usage and failure modes.
As your digital twin covers more and more of the plant’s assets, your ability to predict failure modes and prescribe accurately the required maintenance will be more finely tuned. UReason can help you build and operate digital twins for all your assets.
Fill in the form below for an eBook that explains how we can help you, and click here to have a UReason APM expert contact you for a demonstration.